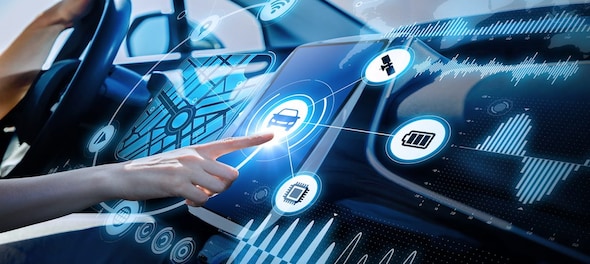
It may take a while to find the JARVIS to your Tony Stark, but real-world machine learning applications are already present in our everyday lives. In India, apps like Aarogya Setu are using artificial intelligence/machine learning (AI/ML) and location tracking capabilities to help the government and frontline workers monitor the spread of COVID-19 and ensure that social distancing norms are followed. According to PWC, global adoption of AI has been accelerated due to the pandemic, and organisations across sectors have embraced AI in a more definitive manner because it has become more of a business necessity than a ‘good-to-have’ solution. The financial services sector alone has witnessed an 82 percent increase in the adoption of AI to navigate the increased business uncertainties and disruptions.
Realising the promise of AI/ML calls for organisations to scale its use enterprise-wide and promote the culture of an insights-driven organisation. Additionally, data is the fuel that keeps machine learning (ML) going, making it important to have a data strategy that works for the business and effectively supports ML applications.
Better data for a better ML experience
A machine learning tool becomes more accurate as it gets fed with more data. Considering that the world will continue to generate vast amounts of information as more people and cities around the world are virtually connected, there is and will be a lot of information for ML tools to work with and learn from. This also implies that organisations need to improve their current in-house data management capabilities to accurately feed the information to ML tools that need to be trained. For this, it will be pertinent to centralise data stored across silos and ensure a uniform treatment so that data can be processed across several platforms, such as on-premises, private or public cloud.
Additionally, professionals who are adept with AI/ML applications will be highly sought after by organisations seeking to become insights driven. Especially with the rise of 5G and the Internet of Things, organisations that need to manage data in motion (i.e., streaming data, data in transit) and data in rest (i.e., data in storage and databases) will require talents who have the knowledge as well as the practical experience of fronting AI/ML projects. Streaming data is particularly important for machine learning systems running on the edges of the networks as those connected devices will decide whether or not to act, based on real-time insights and recommendations.
Democratizing data is another exercise that will encourage more people across an organisation to make informed decisions. This step will be an important one if firms want to counter the dearth of qualified professionals. It may not entirely nullify the need for a data expert but it will train people to effectively function with self-serving platforms and low-code, no-code models. An overarching data governance framework ought to helm the use of this data judiciously to ensure that information shared across teams is accurate and consistent, and data is not misused by unauthorised people.
One enterprise data cloud to govern them all!
Data quality is a fundamental element to successfully train ML models as insights generated from machine learning systems are only as reliable as the quality of the data fed into it. Overarching this use, effective data governance practices will help firms successfully scale machine learning across the organisation. By ensuring that data meets a certain standard – such as being accurate, timely, and relevant—data governance empowers users across the enterprise to make informed decisions. It also subsequently reduces the risk of falling prey to security breaches, and it helps organizations to comply with data privacy regulations and Know Your Customer (KYC) obligations.
With data spread across different platforms, organisations might struggle to effectively enforce data governance using traditional or point data management solutions. An enterprise data cloud can help as it provides an end-to-end, connected data lifecycle solution—from collection to enrichment to reporting to serving to prediction—that can run across a multi- and hybrid cloud environment. It also offers an integrated set of security and governance technologies built on metadata to deliver persistent context across all analytic functions. With context, organisations can ensure data access while being assured that data use is always authorised, tracked, and audited.
It may not be long till machine learning becomes a norm in India. Recognising the potential for AI/ML in transforming economies, the Indian government has mandated policy think-tank NITI Aayog to establish a national program on AI, with a view to guide the research and development in new and emerging technologies. However, poor data quality and lack of access to timely and relevant data can prevent organisations from unleashing machine learning’s full potential. By leveraging an end-to-end, connected data lifecycle solution such as an enterprise data cloud, organisations can confidently scale machine learning across the enterprise to gain timely and reliable insights that will help them unlock value from their data.
—The author, Piyush Agarwal is SE Leader, India, Cloudera. The views expressed are the author's personal
(Edited by : Ajay Vaishnav)
Check out our in-depth Market Coverage, Business News & get real-time Stock Market Updates on CNBC-TV18. Also, Watch our channels CNBC-TV18, CNBC Awaaz and CNBC Bajar Live on-the-go!


Over 50 onion farmers detained in Nashik ahead of PM Modi's visit
May 16, 2024 11:14 AM
Why Google CEO is cautiously optimistic about the election year
May 16, 2024 9:51 AM
Mark Mobius reveals how markets will react if NDA wins 400+ Lok Sabha seats
May 15, 2024 8:09 PM