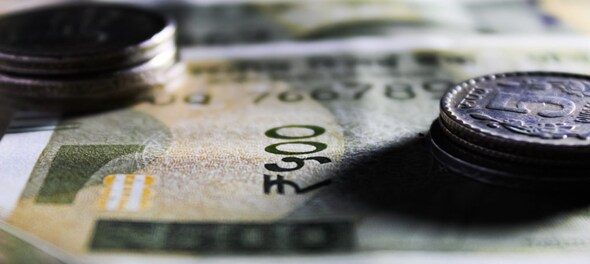
At the height of the pandemic, the fiscal and monetary authorities of India announced a slew of measures to help those impacted. The most important of these measures were moratoriums and restructuring opportunities to help bridge the cash flow disruption caused by lockdowns and general business dislocation.
Moratoriums and restructuring gave breathing room for all borrowers including those stressed even before and irrespective of the crisis. As the moratorium measures wind-down, there is likely to be a significant uptick in bad loans.
As per the RBI financial stability report released in July 2021, macro stress tests indicate that the gross non-performing asset (GNPA) ratio of SCBs may increase from 7.48 percent in March 2021 to 9.80 percent by March 2022 under the baseline scenario; and to 11.22 percent under a severe stress scenario.
With the current economic climate continuing to remain uncertain, financial institutions need to be able to distinguish bad credit from credit needing liquidity support to tide over. Financial institutions also need to be able to identify fraud and fund diversions early. The profitability of financial institutions and in general, stability of the financial system, is predicated on early identification and quick remediation of warning signs in the credit book. This job at hand requires them to have the right set of tools and processes to be able to separate bad loans from borrowers needing liquidity support. The key is not just to be aware that there is impending stress in the loan book but also be able to look through the depth of the problem at hand.
Financial institutions need to upgrade or invest in tools for early warning signals (EWS) to surmount this challenge. From reactive credit risk indicators to models that look through cash flows and predict losses, from financial ratios to machine learning models that identify fraud and fund diversions, EWS tools need to come of age. Separating the wheat from the chaff is a challenge that requires financial institutions to both upgrade their technology and thought process in relation to EWS.
Slice and dice your borrowers into multiple asset classes to assess impact of credit factors
Traditionally borrowers have been classified into asset groups only on the basis of either their product attributes, industry sector or demographics. However, recent times have shown us that those borrowers that may have not have similarities in these seemingly obvious characteristics may still behave in a cohesive fashion. Be ready to monitor the portfolio by multiple asset classes to gain insights and warnings into borrower behaviour.
Leverage the power of data and do not limit it to internal or organisational data sources only
With the advent of web scraping techniques, APIs and open data frameworks, the universe of data sources from bureau data to alternative data sources, social media feeds, news events and macro-economic data have become easily available. While these have always existed, the ease of use to ingest these datasets in the EWS tools combined with borrower-specific data, helps in generating on-demand, trigger based and predictive insights which were largely difficult to come by.
Invest in constant learning, un-learning, and re-learning for credit factors and associated EWIs
Using the regulatory framework on the EWS as a base, there is a need to build a rich repository of credit factors and early warning signals) which cover both qualitative and quantitative methods of identifiers across demographic, behavioural, operational, financial, managerial, and environmental factors. Using metrics like the EWI hit ratio, asset group performance and remediation/ response action performance, the built in models of an EWS can go through a constant calibration process to improve prediction accuracy.
Back-test credit risk and rating models with EWS history and outputs
Credit risk and rating models have traditionally been the gatekeepers of credit flow. The gatekeepers have rarely performed their job as the theory of financial ratios overpowered the obvious fallacies in cash flow projections. EWS provide the opportunity to challenge the gatekeepers and tune their parameters to prevent bad credit from being let into the door.
Don’t get distracted by macros
Macroeconomic indicators might be good to establish and reinforce trends, but each credit turns good or bad on its own merit. The traditional approach of using macros to indicate stress and pumping big data to reinforce a hypothesis only helps increase errors of bias. EWS must be able to predict likely outcomes based on specific business model and cash flow patterns of each borrower and facility.
EWS tools have never been more important for financial institutions. Yet, financial institutions risk re-running old models and old indicators to get vague plausible answers to identify credit stress instead of seeking specific answers. EWS tools need to scale up beyond generics. Otherwise, the genuine moratoriums and restructurings will be clubbed with bad credit and defeat the whole purpose of regulatory and fiscal forbearance.
The author, Viraj Shah, is leader at Business Solution, Acies. The views expressed are personal
First Published: Aug 3, 2021 4:11 PM IST
Check out our in-depth Market Coverage, Business News & get real-time Stock Market Updates on CNBC-TV18. Also, Watch our channels CNBC-TV18, CNBC Awaaz and CNBC Bajar Live on-the-go!


In Ayodhya, voters talk of a promise fulfilled and yearning for development
May 17, 2024 2:10 PM
Fight of heavyweights in Sambalpur where farmers, weavers hold the key
May 17, 2024 12:25 PM
Odisha: Fight of heavyweights in Sambalpur where farmers, weavers hold the key
May 17, 2024 10:22 AM
Lok Sabha Election 2024: What rural Delhi wants
May 16, 2024 10:10 PM